AI and ML Implementation forManufacturing Plant
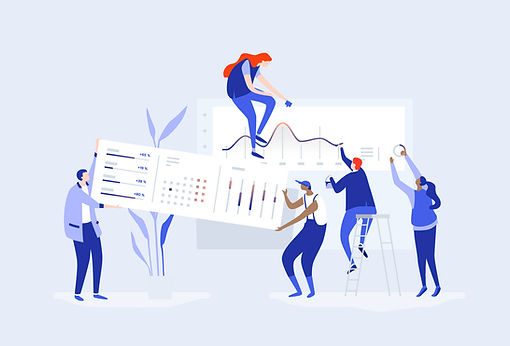
Problem Statement:
Gear Manufacturer of mechanical equipment faced challenges with unexpected equipment failures leading to unplanned downtime. This not only disrupted production schedules but also resulted in higher maintenance costs and reduced overall equipment effectiveness.
Types of Sensors and Integrations Required:
Pressure Sensors
Proximity Sensors
Humidity Sensors
Infrared Sensors
Vibration Sensors
Temperature Sensors
Used to monitor vibrations in machinery. Irregularities in vibration patterns can indicate potential mechanical issues.
Measure the temperature of equipment or surrounding environments. Overheating can signal problems in machinery.
Monitor pressure levels in systems, such as hydraulic or pneumatic systems. Sudden
changes may indicate leaks or malfunctions.
Detects the presence or absence of an object. They can be used to ensure proper alignment or positioning of components in manufacturing processes.
Monitor humidity levels, which can be crucial in certain manufacturing environments to
prevent corrosion or other issues.
Detect infrared radiation, which can be used for temperature measurements or presence detection.
Proccess we Followed:
Data Analysis:
We analyze the data and have some uneven trends.
There is a correlation between room temperature and machine temperature.
Data Preprocessing:
There are some outliers, we used capping techniques to remove it.
We removed the columns that create multicollinearity.
Model Building & Model Evaluation:
We used Random Forest, Gradient Boosting, SVM Model.
We finalized Random Forest as Precision and recall are important, and got a good f1-score.
Deployment:
We deployed a project on AWS EC2, stored data on S3, etc.
Result:
Reduced Unplanned Downtime: The predictive maintenance system significantly reduced unplanned downtime by allowing proactive intervention before critical failures.
Increased Equipment Lifespan: By addressing issues in their early stages, the lifespan of manufacturing equipment was extended, reducing the need for frequent replacements.
Improved Overall Equipment Effectiveness (OEE): The manufacturing process became more efficient, contributing to a higher OEE and increased productivity.
Conclusion:
The implementation of AI-driven predictive maintenance not only addressed the immediate challenges faced by Gear Manufactures but also transformed their manufacturing operations. The proactive approach to equipment maintenance led to cost savings, improved equipment reliability, and enhanced overall manufacturing efficiency.